Healthcare mergers and acquisitions (M&A) sometimes fail – not due to strategy misalignment or lack of capital – but because of overlooked data-related issues. Data is the backbone of every healthcare operation, from patient records and billing systems to analytics and compliance reporting. When that backbone is fragmented, unclean, or insecure, the deal can quickly unravel. Below are the most common reasons why healthcare M&A deals fall apart due to data challenges, with expanded insights for each.
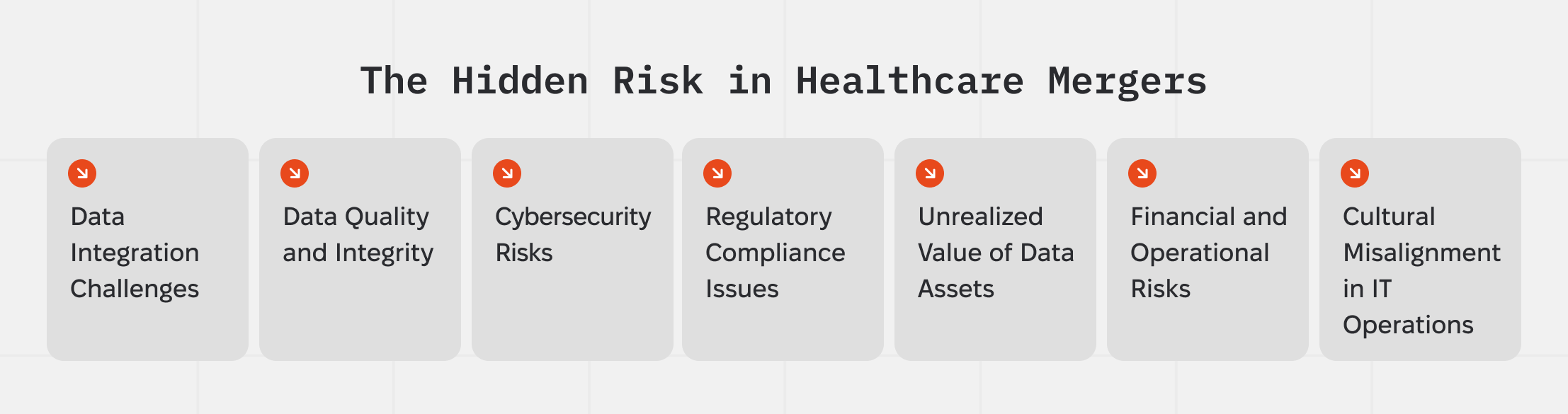
1. Data Integration Challenges
Disparate Systems
Healthcare organizations often operate on a mix of legacy and modern technologies that weren’t built to work together. During a merger, this lack of interoperability becomes a major roadblock. Consolidating electronic health records, billing systems, and care coordination platforms takes time and money – slowing down integration and delaying expected synergies.
Legacy Infrastructure
Outdated systems that come with significant technical debt make integration even harder. These infrastructures are often brittle, lack modern interfaces, and require substantial rework to connect with newer systems. As a result, they increase the overall risk and cost of the deal – and can even be a dealbreaker.
2. Data Quality and Integrity
Inconsistent or Incomplete Data
Poor-quality data is a hidden liability in many healthcare M&A deals. Issues like missing values, inconsistent coding practices, and duplicate records can compromise patient safety, skew analytics, and delay decision-making – undermining the operational effectiveness of the newly merged entity.
Unclean Data Sets
If data hasn’t been standardized, validated, or cleaned before the merger, it becomes an obstacle to just about everything: from accurate reporting and compliance to AI implementation and patient matching. These issues tend to snowball post-merger if not addressed upfront.
3. Cybersecurity Risks
Data Breaches
M&A increases vulnerability to cyberattacks, especially during integration when security protocols are in flux. Healthcare is already the most targeted sector, and data breaches during M&A can be particularly damaging – financially and reputationally.
Regulatory Non-Compliance
If the target company lacks strong security controls, the acquirer may inherit compliance risks. Post-merger audits often reveal weak data protections that could lead to violations of privacy laws like HIPAA or GDPR – resulting in fines, lawsuits, or loss of trust.
4. Regulatory Compliance Issues
Data Privacy Violations
Misalignment in how each organization handles personal health data – such as their roles under privacy frameworks – can create confusion about accountability. These gaps often result in compliance failures if not clearly addressed during due diligence.
Interoperability Standards
Failure to meet technical standards for exchanging health data across systems can delay the benefits of the merger. Lack of interoperability slows down clinical integration, affects care delivery, and prevents data from flowing efficiently across the new organization.
5. Unrealized Value of Data Assets
Overlooked Strategic Potential
Sometimes the full value of a company’s data – its potential for generating insights, building AI models, or improving business performance – isn’t properly evaluated. This oversight can diminish the strategic upside of the acquisition and limit long-term innovation.
Underutilized AI Capabilities
Even when data assets exist, if they’re fragmented, unstructured, or missing key annotations, they’re difficult to use for AI or analytics. As a result, the expected gains in predictive care, automation, or operational efficiency never materialize.
6. Financial and Operational Risks
High Integration Costs
The price of integrating incompatible systems – whether it's EHRs, financial software, or internal workflows – is often underestimated. These costs can consume a large portion of the projected deal value, eroding ROI and slowing progress.
Delayed Care Coordination
Without seamless system integration, care coordination suffers. Critical tasks like referrals, treatment tracking, or billing reconciliation get delayed, which negatively impacts patient experience and the financial performance tied to quality-based reimbursement.
7. Cultural Misalignment in IT Operations
IT Governance Conflicts
When merging organizations have different approaches to data governance – such as who owns what, who has access, and how policies are enforced – alignment becomes messy. These misalignments often slow integration and breed internal resistance.
Change Management Gaps
Even the best tools and processes won’t succeed if the IT teams and end-users aren’t aligned. Without a strong change management plan, new systems go underutilized, workflows get disrupted, and integration momentum stalls.
Healthcare M&A Risk Mitigation Tool Matrix
Data challenges are a common reason healthcare deals stall or underdeliver. From fragmented systems and dirty datasets to compliance blind spots and misaligned governance, the risks are everywhere. But the good news? Most of them are preventable.
This matrix maps the most common data-related pitfalls to specific tools, practices, and roles – plus the caveats you need to know before assuming any checkbox will fix things. Use it to guide your due diligence, integration planning, or simply to keep your team aligned on what actually works (and what doesn’t) in real-world M&A execution.
Conclusion
Healthcare M&A is evolving, and dealmakers face growing pressure to treat data as a core asset. With AI adoption accelerating and regulatory expectations rising, data readiness will increasingly define deal success.
The good news? You can see it clearer. Technical due diligence, AI readiness assessments, and structured integration plans aren’t just nice to have – they’re the difference between a deal that delivers value and one that derails.
For buyers eyeing transformative opportunities in healthtech, biopharma, and medical systems, data fluency will be a competitive advantage. And the firms that treat integration planning, compliance alignment, and AI governance as early-stage priorities will lead.